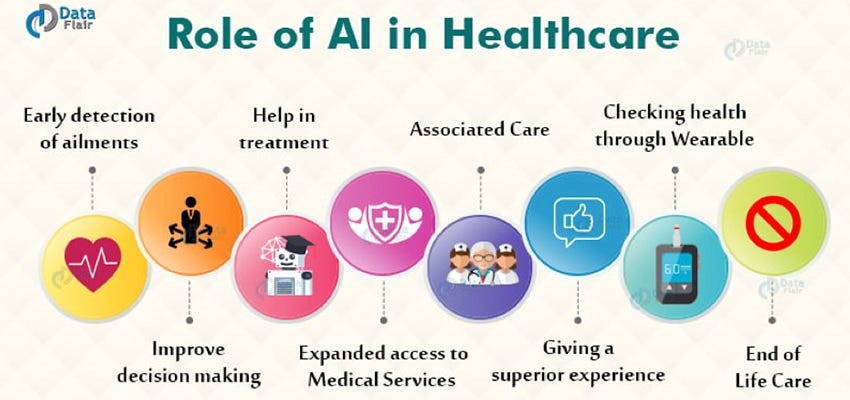
Introduction
Artificial Intelligence (AI) has rapidly transformed the landscape of healthcare and biotechnology, offering breakthroughs that once seemed impossible. However, this technological marvel comes with its share of controversies and challenges. Geoffrey Hinton, often referred to as the “godfather of AI,” recently stepped down from his position at Google, voicing concerns about the potential dangers of this powerful technology. On the other hand, stories like that of a woman in West Sussex, highlight the life-saving potential of AI—her breast cancer was detected by AI after a routine scan initially missed it.
AI has become deeply embedded in our daily lives, influencing everything from how we interact with technology to how we manage our health. For some, it serves as a tool for productivity and innovation; for others, it fulfils emotional voids, with AI companions acting as virtual friends or partners. This duality raises a critical question: how safe and reliable is AI, particularly in healthcare? While AI has revolutionized diagnostics, treatment planning, and drug discovery, it also raises ethical concerns, including data privacy, bias in algorithms, and over-reliance on machine-generated insights. Striking the right balance between leveraging AI’s potential and mitigating its risks is vital as we move forward. This article delves into the transformative role of AI in healthcare and biotechnology, examining its promising applications and the challenges that demand our attention.
The Evolution of AI in Healthcare and Biotechnology
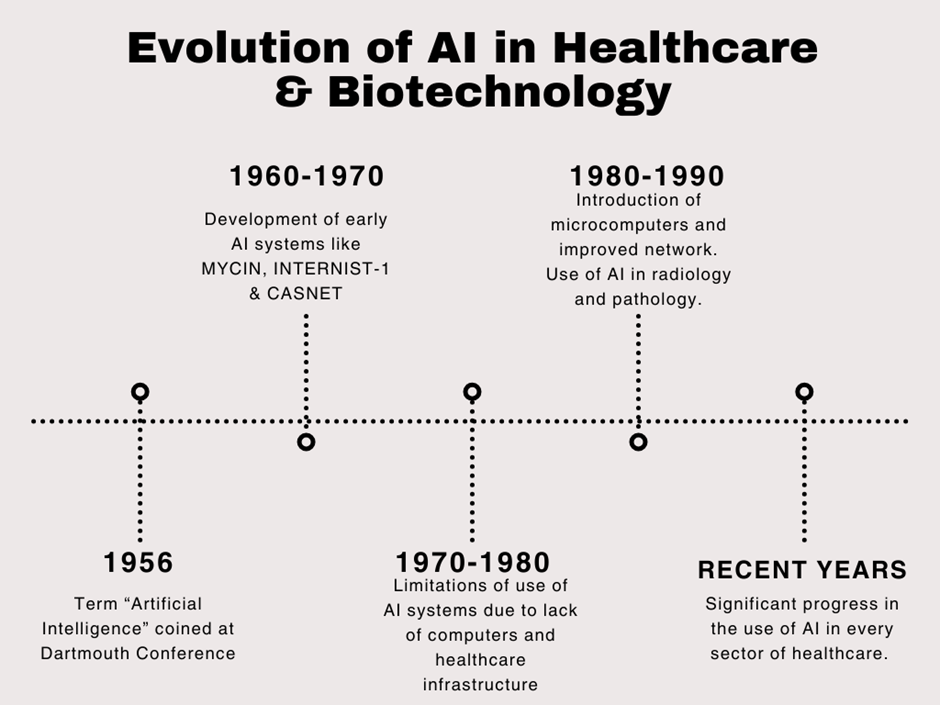
The journey of artificial intelligence (AI) in healthcare began in 1956 at the Dartmouth Conference in New Hampshire, where the term "Artificial Intelligence" was first introduced. From its inception, AI aimed not only to replicate human intelligence but also to address complex challenges across fields like medicine. Early efforts in the 1960s and 1970s led to the development of systems such as MYCIN, INTERNIST-1, and CASNET, designed to aid in diagnosis and treatment. These systems were particularly focused on rule-based decision-making and expert systems to support clinical reasoning. However, these pioneering tools were limited by the computational and infrastructural constraints of their time and failed to achieve routine clinical use.
The 1980s and 1990s saw significant progress with the advent of microcomputers and improved network connectivity, allowing AI systems to process and analyse increasingly complex healthcare data. During this period, AI expanded into imaging and pattern recognition, enabling advancements in radiology and pathology. These advancements provided a foundation for AI systems to process and analyse increasingly complex healthcare data. Despite these improvements, widespread adoption remained elusive due to challenges like data availability, standardization, and clinician acceptance until the recent years.
The Applications of AI in Healthcare and BiotechnologyIn the recent years, several critical advancements have propelled AI's integration into healthcare. The exponential growth in computing power has enabled rapid data processing. Advances in genomic sequencing have also created vast databases that support personalized medicine. The widespread adoption of electronic health record systems has provided structured data for AI applications, and improvements in natural language processing and computer vision have allowed machines to interpret medical text and images with remarkable accuracy. Innovations in deep learning have uncovered patterns in rare diseases. Advancements in precision micro-mechanics have facilitated robot-assisted surgeries.
These developments have culminated in transformative applications of AI in healthcare. From early and precise diagnostics to enhanced medical imaging, AI has revolutionized the detection and treatment of diseases. It has accelerated drug discovery, optimized hospital information systems for better patient management, and supported critical decision-making in emergency care and surgeries. AI has also advanced mental health treatment by enabling early detection and personalized therapy and has played a role in preventive care through nutrition analysis and real-time health monitoring via wearable devices.
Revolutionizing Patient Care Through AI
While AI demonstrates immense potential in healthcare and biotechnology research, its practical impact is equally profound in individual patient care. Two notable examples highlight how AI has reshaped in-hospital dynamics and proven to be a significant asset for patients:
Ø Top of FormCase study: AI in Breast Cancer Detection
Sheila Tooth, a 68-year-old breast cancer survivor, credits artificial intelligence (AI) with saving her life. Reflecting on her experience, she expressed her amazement: “It’s extraordinary. My friends and I can hardly believe AI can detect what the human eye sometimes misses. I feel incredibly lucky.” Sheila underwent a routine mammogram at University Hospitals Sussex NHS Foundation Trust, which uses AI to enhance breast cancer screening. Initially, her scan was deemed clear by human readers, missing a critical cancerous cell. However, AI flagged the anomaly, leading to a diagnosis and timely intervention.Dr. Olga Strukowska, consultant radiologist and director of the West Sussex breast screening program, described the AI results as “exciting,” emphasizing that earlier and more precise detection significantly improves patient survival rates. University Hospitals Sussex is one of 15 trusts in the UK participating in a pioneering project integrating AI into mammogram analysis. Building on the promising outcomes, the trust plans to join a national randomized controlled trial, advancing the next phase of AI adoption in breast cancer screening.
Ø Case Study: Enhancing Cancer Treatment with AI
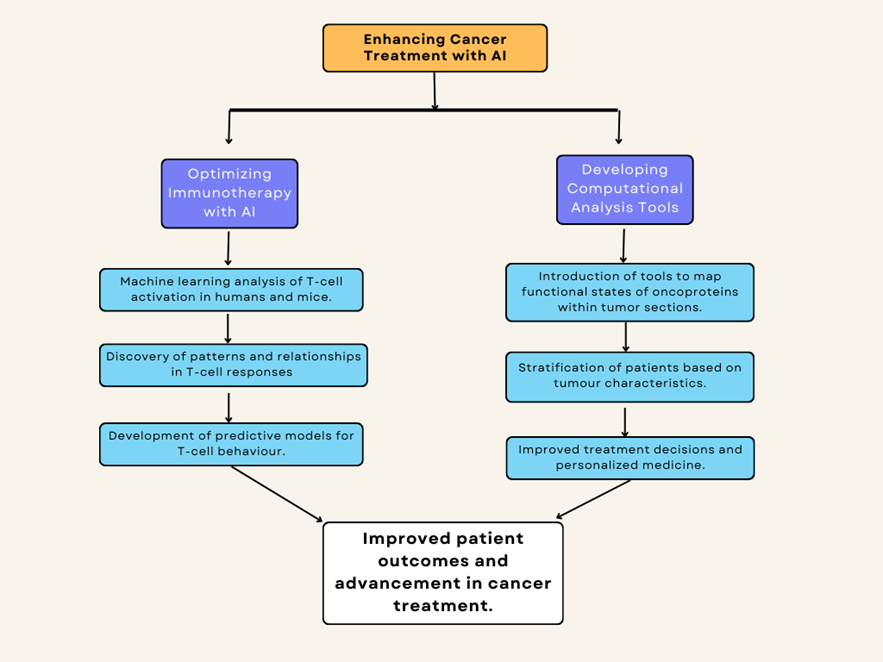
Artificial Intelligence (AI) has become a powerful tool in advancing cancer treatments, offering insights that traditional methods often cannot achieve. A compelling example comes from researchers at the National Cancer Institute (NCI) and their collaborators, who harnessed AI to optimize immunotherapy, a treatment designed to empower the immune system to fight cancer.
The researchers applied machine learning to analyse vast datasets of T-cell activation in humans and mice. This analysis revealed patterns and relationships that were previously undetectable. By understanding how T cells—key players in the immune response—behave when interacting with tumours, the team developed predictive models to forecast T-cell responses. This insight is crucial in tailoring immunotherapies, ensuring they are more effective and patient-specific.
In addition, the researchers introduced a computational analysis tool capable of precisely mapping the functional states of oncoproteins within tumours sections. This tool enables clinicians to better stratify patients based on the unique characteristics of their tumours, paving the way for personalized medicine. By offering a clearer understanding of tumour biology, clinicians can make more informed treatment decisions, ultimately improving patient outcomes.
The Drawbacks of AI in Healthcare: Can We Trust it?
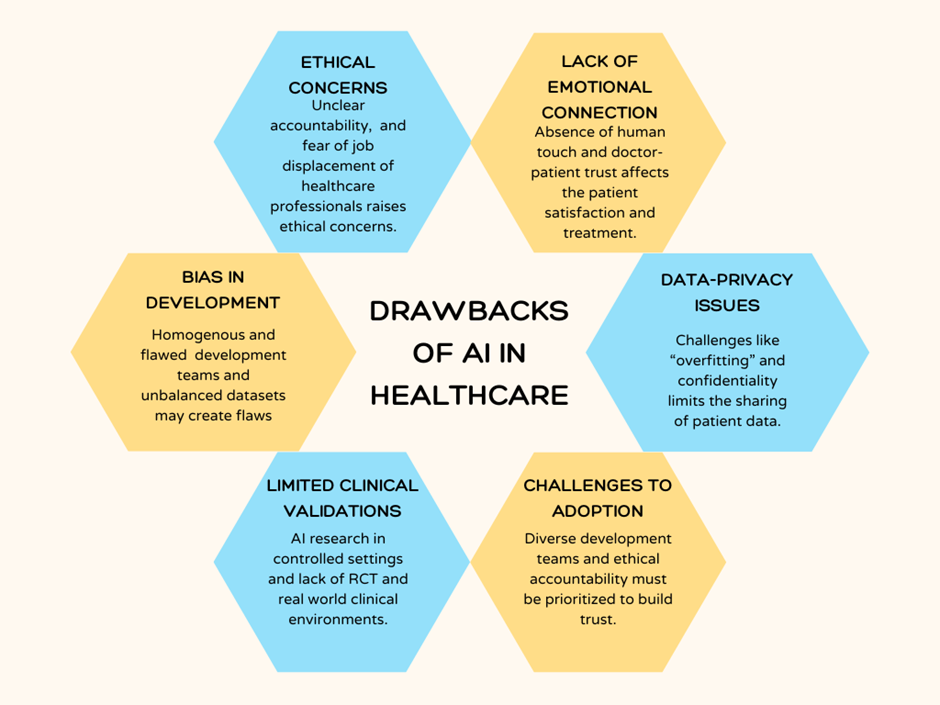
While artificial intelligence (AI) has shown immense promise in revolutionizing healthcare, it is not without significant challenges and drawbacks that raise questions about its reliability and suitability for complete reliance. A key concern is the absence of the emotional connection central to the doctor-patient relationship. Trust, empathy, and understanding form the cornerstone of effective medical care, and these human elements cannot be replicated by algorithms. The lack of such bonds could compromise patient satisfaction and adherence to treatment.
Data-related issues also present substantial hurdles. Patient records are highly confidential, and the reluctance of institutions to share such data hampers the development of robust AI models. Additionally, biases in data collection can skew AI outcomes. Underrepresentation of minorities in datasets, for instance, may lead to discriminatory or inaccurate predictions, potentially exacerbating health inequities. While creating multi-ethnic datasets can mitigate some biases, issues such as ‘overfitting’ where AI learns irrelevant correlations remains challenging to address.
Ethical concerns further complicate AI’s role in healthcare. Accountability for AI-driven decisions, especially in life-or-death situations, is a pressing issue. Determining who among the developers, clinicians, or institutions will bear the responsibility for poor outcomes remains unresolved. Moreover, fears of job displacement by AI systems contribute to scepticism and resistance among healthcare professionals.
Another major drawback is the limited clinical validation of AI technologies. Most AI research in healthcare has been conducted in controlled environments rather than real-world clinical settings. This lack of practical data makes it difficult to generalize findings and undermines confidence in AI’s effectiveness in improving patient outcomes. Randomized controlled trials, the gold standard in medicine, have yet to provide definitive evidence of AI’s benefits in healthcare.
Bias is also ingrained in the development process. Homogeneous development teams and unbalanced datasets can embed cultural and technological prejudices into AI systems, resulting in flawed tools that may inadvertently perpetuate inequities.
Given these challenges, AI in healthcare must be approached with caution. While its potential is undeniable, achieving widespread trust and adoption will require addressing these drawbacks through better data practices, diverse development teams, rigorous clinical validation, and a commitment to ethical accountability.
ConclusionArtificial Intelligence has undeniably transformed the healthcare landscape, enabling breakthroughs in diagnostics, treatment personalization, and drug discovery. Its ability to process and analyse vast amounts of data offers hope for addressing some of medicine's most complex challenges. However, the road to widespread adoption is fraught with obstacles. Issues such as data privacy, algorithmic bias, ethical accountability, and the lack of clinical validation must be addressed for AI to achieve its full potential.
AI should not be seen as a replacement for human expertise but as a tool to complement it. Striking a balance between leveraging its capabilities and maintaining the human touch in medicine is essential. With thoughtful implementation, diverse development teams, and rigorous validation, AI can become a cornerstone of modern healthcare. As we move forward, the focus must remain on responsible innovation—ensuring that the benefits of AI are equitably distributed while minimizing risks and maintaining trust in this transformative technology.
References:
https://www.bbc.com/news/world-us-canada-65452940
https://www.bbc.com/news/articles/cd9ndpdy0q3o
https://pmc.ncbi.nlm.nih.gov/articles/PMC8285156/